Coral collection and sampling
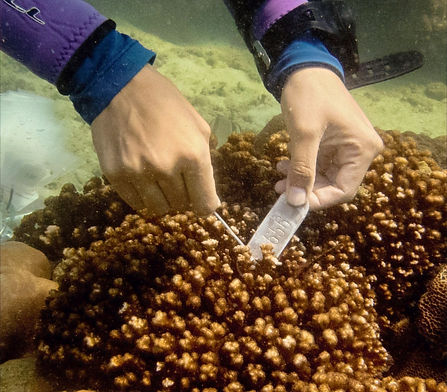
I'm a paragraph. Click here to add your own text and edit me. It's easy.
DNA and RNA extractions
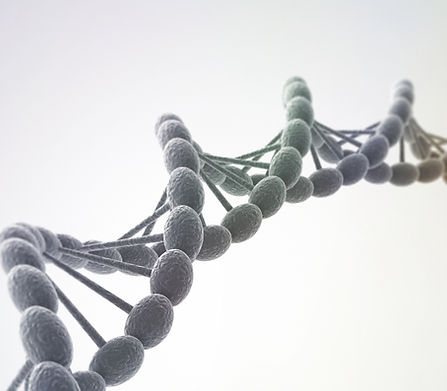
In the Reef Futures Lab we use a DNA extraction protocol developed to optimize extraction of the Symbiodiniaceae algae. However, this protocol is time consuming.
Coral physiology

-
Chlorophyll fluorescence:
​
-
Blasting of coral tissue
​
-
Chlorophyll-a content
​
-
Symbiodiniaceae areal estimation (Microscopy)
​
-
Calcification rates using the buoyant weight technique
​
-
Total lipid estimation
Data analysis
Most of my current data analysis uses R and R packages. During college, I first used proprietary programs for statistical analysis. The learning curve was not so steep, but replicating the analyses became difficult after changing computers or losing access to the software licences (They were quite expensive for a student!). Additionally, using these programs became more difficult when I changed my operating system to a GNU/Linux distribution that was more according to my philosophy.
​
I finally decided to stop being scared of R and tried it during my first year in grad school. Yes, it took me more time to learn how to start using it, but now I cannot go back to any other software for my statistical analyses and graphics. R has thousands of packages, so you have almost limitless options.
R also makes it easier to do reproducible and open science. It is free, runs on UNIX, Windows and MacOS, and if you are good keeping scripts that are well documented and organized, anyone could reproduce and validate your analyses! I personally like the R (for statistics and graphics), Markdown (for nice code documentation), git (for version control) and GitHub (for hosting/sharing/collaboration) combo.
​
I found that learning R and the nuances of its packages was easier when I was running my own analysis. I suggest to get familiar with the basics and then jump into your own work even if it seems complicated.
​
Here are some useful links and materials to get started:
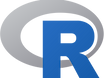


-
R:
-
R manuals in multiple languages
-
R studio offers a graphical user interface
-
R packages will help you to develop good R coding practices. Also has a chapter on using RStudio, Git and GitHub together.
-
​
-
Git and GitHub:
-
Pro Git book by Scott Chacon and Ben Straub. I followed this step by step when I was learning to use git. Highly recommended
-
​
-
Markdown:
​​
-
Integration:
-
R and git: Using Git with RStudio
-
Markdown and GitHub​ (Writing on GitHub)
-
This is an example of data analysis in which I integrated these tools to make the project reproducible:
​
-
Data analysis for the paper Coral reef resilience to thermal stress in the Eastern Tropical Pacific (doi:10.1111/gcb.15126)
-
GitHub repository with R scripts, data, and ReadMe file
-
Repository output generated with Markdown and Knit
-
Repository archive in Zenodo that corresponds with the last version of the data published​
-
Protocols
-
protocols.io: A secure platform for developing and sharing reproducible methods
Paper access
-
Unpaywall (database and browser extension): links to full-text articles from open-access sources. The content is harvested from legal sources including repositories run by universities, governments, and scholarly societies, as well as open content hosted by publishers.
​​
-
OpenAccessButton: a browser extension that finds free, legal, full-text articles in aggregated repositories in the world, hybrid articles, open access journals, and authors' personal pages.